ML Workshop
International Workshop on
Machine Learning in Astronomy (Hybrid)
Organized by
National Center in Big Data and Cloud Computing, NED-UET, Karachi, Pakistan
In collaboration with
Department of Astronomy, Tsinghua University, Beijing, China
&
Radio Galaxy Zoo: EMU Citizen Science Collaboration
November 22-23, 2023
At
National Center in Big Data and Cloud Computing, NED-UET, Karachi, Pakistan
The primary objective of this workshop is to provide the participants with practical insight of the utilization of machine learning algorithms in a variety of astronomical research domains.
Live-Streaming: Via Zoom and YouTube - link will be available soon
Registration: To register fill the registration form.
- · There is no registration fee to attend this workshop.
- · Registration is mandatory and seats are limited for both in-person and online participants
- · Participation certificates will be issued to the registered participants on full attendance during the workshop.
Registration Deadline: November 15, 2023
Approved in person participants notification: November 20, 2023
For any queries or questions, please feel free to contact us at ncbc@neduet.edu.pk
Tentative Speakers
Dr. Andreas is an assistant scientist (assistant professor equivalent) affiliated with the Infrared Processing and Analysis Center at Caltech. His research focuses on the formation of galaxies in the early universe and the evolution and ultimately death of massive galaxies at later epochs in cosmic time. He reviews articles for prestigious astronomy journals (MNRAS, ApJ, A&A, and Nature Astronomy) on a regular basis. Moreover, he serves on proposal reviewing committees such as for Hubble and the Canadian Time Allocation Committee (CanTAC). He is co-founder of the IPAC Visualization Group (IViz), a think-tank for advanced data visualization.
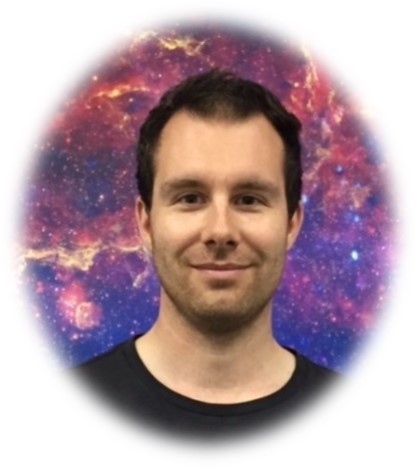
Talk Title: Visualization with unsupervised Machine Learning
Abstract: Big Data is now omnipresent in astronomy. Machine learning algorithms are the ideal tool not only for computations, but also for visualization. In my lecture, I will show how to use unsupervised machine learning methods (such as SOM and T-SNE) to visualize correlations in N-dimensional catalog and imaging data sets.
Dr. Dario is a postdoctoral researcher working at the Max Planck Institute for Radio Astronomy (Bonn, Germany) since 2015. Between 2014-2015, he worked as a postdoctoral fellow at the University of Alberta (Edmonton, Canada). He obtained my PhD from the Heidelberg University (Heidelberg, Germany) for which he worked at the Max Planck Institute for Astronomy on the "Gas organization in M51 - The impact of spiral arm dynamics on Giant Molecular Cloud properties" topic. He obtained my MSc in Astrophysics and Space Physics and BSc in Physics from the University of Milano-Bicocca (Milano, Italy).
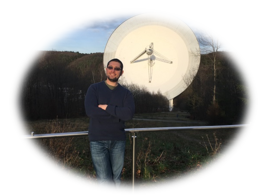
Dr. Dario’s research focuses around Molecular clouds, Milky Way structure, galaxy evolution, star formation quenching, single-dish and interformetric mm/cm observations, machine learning.
Talk Title: Recognizing patterns in the sky: a brief journey across machine learning tools for star formation and galaxy analyses
Dr. Rafeel obtained his doctoral degree in astrophysics from the Institute of Space & Planetary Astrophysics (ISPA), University of Karachi, Pakistan in 2016. He completed two postdoctoral projects (including the Fondecyt Postdoctorado) at the Department of Astronomy, University of Concepcion, Chile during 2017 – 2021. Currently he is working as associate professor at the Center of Investigation in Astronomy (CIA), University of Bernardo O’Higgins, Santiago, Chile..
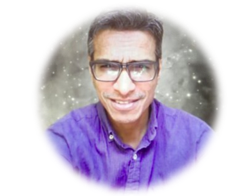
Talk Title: Morphology of the circumstellar disc in YSOs
Abstract: Circumsteller disc around young stellar objects (YSOs) is an interesting astrophysical phenomenon and still poses challenges to be fully understood both observationally and numerically. This study of mine, which is based on computational modelling explores two distinct turbulent regimes – subsonic Kolmogorov and supersonic Burger regimes using Smoothed Particle Hydrodynamics (SPH) method with a computer simulation code GRADSPH. It reports that massive circumstellar discs are more commonly formed in the subsonic Kolmogorov regime compared to the supersonic Burger regime. Both turbulent regimes result in the formation of circumstellar discs, mostly with a radius of approximately 15 astronomical units (au). However, the Kolmogorov regime tends to produce a greater number of extended discs with radii exceeding 15 au. In general, both turbulent regimes yield circumstellar discs with a range of sizes, spanning from 7 au to 30 au in the Kolmogorov regime and from 13 au to 39 au in the Burger regime. Similarly, the disc mass ranges differ between the two regimes, with a higher maximum disc mass in the Kolmogorov regime. The disc-to-stellar mass ratio (Mdisc/Mstar ) is reported to be higher in models of the Kolmogorov-type turbulence compared to the Burger-type turbulence. The study did not find any correlation between disc radius (Rdisc ) and disc mass (Mdisc ) across the explored range of initial temperatures (8 K to 14 K) and the type of turbulence. The radial profiles of circumstellar discs do not appear to correlate with the initial conditions prevailing in the prestellar gas core (PGC). Misaligned discs with respect to the rotational axis of the PGC are also very common.
Dr. Richard Grumitt is a Shui Mu Fellow at Tsinghua University, specializing in the development of computational methods for Bayesian inference, and their application to cosmological inference problems. His recent work has focused on leveraging normalizing flows to accelerate approximate inference algorithms, and in developing hierarchical Bayesian models for cosmological component separation. He previously completed his PhD at the University of Oxford, where he worked on component separation for cosmic microwave background polarization observations
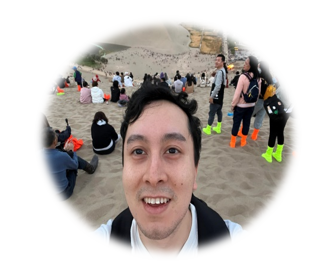
Talk Title: Bayesian Inference: Computational methods and applications to cosmology and astrophysics
Abstract: Bayesian statistics has been the workhorse of many astrophysical and cosmological analyses. Its practical application has typically involved the use of Markov Chain Monte Carlo (MCMC) algorithms. In this workshop I will cover the fundamentals of MCMC methods, before moving to survey the current state-of-the-art for performing Bayesian inference with high dimensional and expensive models. We will finish the workshop by running real cosmological inference problems using pocoMC, a state-of-the-art package exploiting normalizing flows for gradient-free inference.
With an extensive track record spanning over a decade, Dr. Oozeer brings a wealth of international experience as a seasoned Data Scientist, a journey that began with his groundbreaking PhD project in Mauritius. Throughout his career, he strategically partnered with global enterprises, playing a pivotal role in fostering the growth of data-centric organizations. His proficiency encompasses a wide spectrum, encompassing Machine Learning, Data Science, Product Management, Agile Methodologies, and Software Engineering
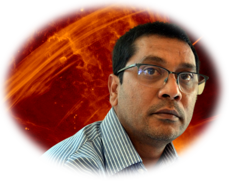
Beyond technical prowess, his leadership acumen shines through in his adeptness at overseeing and mentoring graduate, post-graduate, and doctoral candidates, as well as dedicated staff within the South African radio Astronomy Observatory (SARA).
One of his standout achievements lies in spearheading and orchestrating groundbreaking data science workshops across the African continent. These pioneering initiatives not only stand as first-of-their-kind endeavors but also manifest in tangible results, as evidenced by securing millions in funding from international backers—a testament to my impactful contributions in bridging resources and expertise to Africa's shores.
Talk Title: Radio Frequency Interference: A data scientist view of peta-bytes of “junk”
Abstract: In our unrelenting quest to unravel the mysteries of the cosmos, we radio astronomers are embarking on the construction of increasingly sensitive radio telescopes, exemplified by groundbreaking instruments like MeerKAT, ASKAP, and the future Square Kilometre Array (SKA). However, this remarkable progress carries with it a caveat – the acquisition of a multitude of extraneous signals, commonly known as radio frequency interference (RFI), which taint the very essence of peta-bytes of scientific radio data.
In this talk, I shall elucidate how a profound comprehension of the RFI landscape can empower astronomers to glean deeper insights from their radio datasets, consequently furnishing them with the assurance to accurately identify and mark their data. I will show how probabilistic models have been used to understand the RFI environment from analysis of around 2 peta-bytes of observational data.
Dr. Eleni Vardoulaki is an astrophysicist with a doctorate from the University of Oxford, and a postdoctoral researcher at the Thüringer Landessternwarte Tautenburg. She is member of three large international collaborations, COSMOS, EMU, MeerKAT–MIGHTEE, and LOFAR. She is also member of the SKA Continuum and the ngVLA Science working groups. She is also the chair of the working group Radio Galaxy Zoo 2 – EMU. She is the lead editor and author of an edited Springer volume on ‘Data-Intensive Radio Astronomy’. She is the principal investigator (PI) for COSMOS LOFAR DDT observations, and the co-lead of the citizen science project ‘Radio Galaxy Zoo – EMU’.
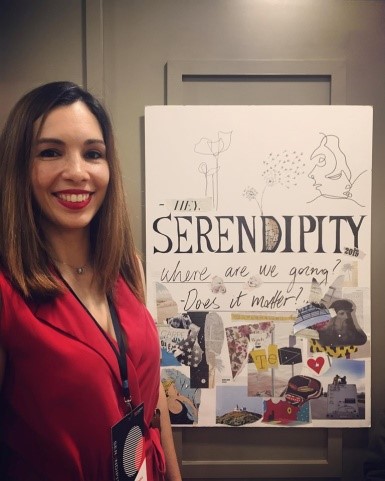
Dr. Eleni’s research is oriented around active galaxies and their relation to their environment, and how galaxies grow and evolve throughout the Universe. I am the PI of the COSMOS LOFAR DDT, LOFAR2.0 large project and the future LOFAR2.0-Greece station. She is a TEDx speaker, and also the founder (9/2018) and manager (9/2018-6/2020) of Astronomy on Tap Bonn.
Talk Title: Conventional classification methods, robust training samples, and hands-on examples
Abstract: The radio sky is filled with a multitude of radio structures, ranging from circular, ellipsoidal, elongated, string-like shapes, to more amorphous and complex entities. The nature of this radio emission is related to physical properties linked to the nuclear activity or star-forming processes of distant galaxies. To characterise the observed radio emission and associate it to host galaxies we use multi-wavelength observations of the same sky area. Conventional methods employ simple automatic algorithms and visual inspection techniques to create robust samples and catalogues. These can eventually be used to train deep-learning algorithms, used for classification of millions of radio sources. I will give real examples for source identification and classification using the multi-wavelength dataset of the COSMOS field and I will introduce the Radio Galaxy Zoofor the Evolutionary Map of the Universe Survey (RGZ-EMU) citizen science project. Hands-on examples using the RGZ-EMU platform will follow.
Dr. Hongming is an astrophysics researcher, science communicator and astro-machine learning educator. He is now working as a Shuimu postdoctoral research fellow at the Department of Astronomy, Tsinghua University. His research focuses on applying machine learning on hunting rare radio galaxies, identifying radio galaxies of diverse morphologies and investigating under what circumstances one would believe the astronomy predictions given by machine learning algorithms.
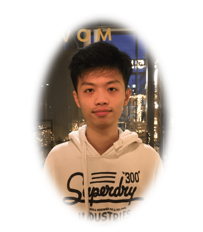
Talk Title: Radio Galaxy Zoos: How machine learning incorporates with citizen science
Abstract: While citizen science and conventional human visual inspection has been proved to be useful for source finding and morphology classification, survey cataloguing in the big data era can hardly be efficiently handled by citizen science itself only. In this talk, I will introduce how Radio Galaxy Zoo team combine machine learning and citizen science to handle survey cataloguing challenges such as source finding and classification, unusual object identification. I would also mention the role of machine learning in Radio Galaxy Zoo: EMU, the latest RGZ offshoot.
Muhammad Ali Ismail, PhD, Senior Member IEEE & MIET is a Professor at Department of Computer and Information Systems Engineering, NED University of Engineering and Technology. He is also serving as Director High Performance Computing Center and Scientific Director National Center in Big Data and Cloud Computing at same University. He has more than 20 years’ experience of research, teaching and administration in both national and international universities. He has published over 75 scientific papers in international journals and conferences along with U.S. patent. He has won many of the national and international grants of worth above Rs. 200 Million. He is also the recipient of Research Productivity Award by Pakistan Council for Science and Technology- Ministry of Science and Technology, Government of Pakistan. His current research interests include High Performance Computing, Computational Astrophysics, Big data mining, Cluster and Cloud Computing, Multicore processor architecture and programming, Machine learning, Heuristics and automatic design space exploration.
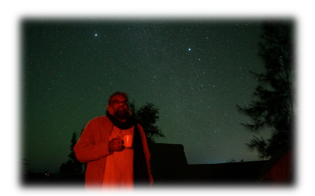
Talk Title: Intelligent sunspots detection and forecasting using advanced Machine learning
Dr. Syed Faisal Ur Rahman has a PhD in space sciences and a degree in Engineering. He has more than 15 years of experience in industry and academia. He is currently serving as CTO of Blockchain Laboratories LLC, Wyoming, USA. He is also actively involved in Radio Astronomy and Cosmology research. He is a member of two Australia based international collaborations, EMU-ASKAP and WALLABY-ASKAP Radio Astronomy surveys.
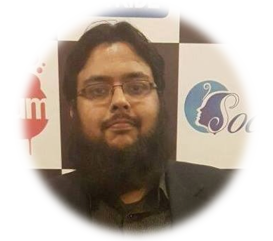
Talk Title: Clustering statistics for cosmology
Abstract: In this two-part workshop, we will dive into the realm of clustering statistics and its pivotal role in advancing our understanding of cosmology. The first session will introduce participants to Angular Auto-Correlation Functions (ACFs) and Cross-Correlation Functions, illustrating their significance in probing large-scale structures in the universe. We will explore how these statistics are instrumental, especially when applied to large scale surveys like RACS, NVSS, EMU, 2MASS and others.
The second session will be a hands-on lab, providing participants with the opportunity to employ Python-based treecorr to calculate ACFs and cross-correlation functions. Through practical exercises, attendees will gain firsthand experience in utilizing these statistical tools for cosmological research. This interactive session aims to empower participants with practical skills that they can apply in their own research endeavors.
Ms. Xinyue is a doctoral researcher working on machine learning applications on transients, especially Superluminous Supernovae and Tidal Disruption Events.
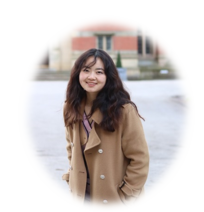
Talk Title: Find Needle in the haystack
Abstract: Known for their efficiency in analyzing large data sets, machine learning-based classifiers have been widely used in large sky survey pipelines. The upcoming Vera C. Rubin Observatory Legacy of Time and Space Survey (LSST) will generate millions of real-time alerts every night, and identifying unusual or interesting transients in their early stages will greatly help researchers study their evolution.
Ordinary classifiers only utilize image, light curve, spectrum, or host information, while multidimensional classifiers utilizing combined information are able to learn the connections between them, thus providing better accuracy. Using ~6000 transients from the ZTF Bright Transient Survey as training and testing data, we develop a novel hybrid CNN+DNN classifier (NEEDLE) that recognizes superluminous supernovae, tidal disruption events and normal supernovae by using their cutouts of the detection and reference images, simple photometric information contained directly in the alert packets, and host information from PanSTARRS. The averaged accuracy reaches 77% (SNe), 73% (SLSNe-I) and 60% (TDEs) in the test set with 15 objects for each class. Our network is designed with LSST in mind and we expect performance to improve further with the higher resolution images and more accurate host photometry that will be available from Rubin.
Ms. Jiani Chu is a graduate student in Tsinghua University, she is interested in galaxy evolution and machine learning. She got her undergrad degree in physics at Zhejiang University.
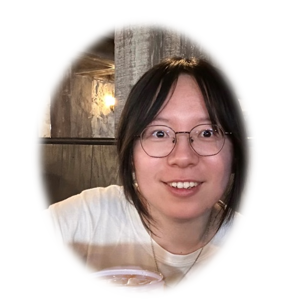
Talk Title: Galaxy mass stellar and total estimation using machine learning
Abstract: Conventional methods for estimating galaxy mass suffer from model assumptions and degeneracy. Machine learning, which reduces the reliance on such assumptions, can discern to what extent present observations can yield predictions for the distribution of stellar and dark matter. In this talk, I will talk about using machine learning to estimate dynamical property of TNG galaxies. I use multi-branch ResNet to predict galaxy masses and mass-to-light ratio using galaxy multi-band images and velocity maps as input, and use GBDT to investigate which global feature contributes to the prediction.
Ms. Shiyu Yue is a senior undergraduate from Sun Yat-sen University, currently serving as a student RA at the Laboratory for Space Research in the Hong Kong University. Her research revolves around cosmic statistics and the application of XAI in radio galaxy classification.
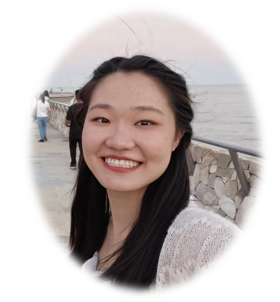
Talk Title: “May I trust you?”: Explainable AI for Radio Galaxy Classification
Abstract: In this recorded lecture talk, I will introduce you to an explainable AI technique: Local Interpretable Model-agnostic Explanation (LIME), and run through how we used LIME for interpreting model prediction rationales of a CNN-based radio galaxy classification algorithm. A Jupyter notebook tutorial will be provided along with the recorded talk.
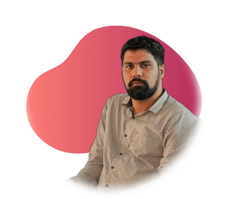
Mr. Uzair Abid is an experienced Data Scientist with a wealth of knowledge and an unyielding passion for data-driven exploration, he holds a Master's degree specializing in Data Engineering from NED University of Engineering and Technology and a Bachelor's degree in Software Engineering. His expertise spans a wide spectrum of technical domains, including Computer Vision, Big Data Analytics, Machine Learning, Deep Learning, Transfer Learning, NLP Generative AI, Databases, and Web Engineering. Throughout his career, he remained committed to robust engineering practices, which has well-prepared me to navigate the complexities of data landscapes. In his current role as a Team Lead at the National Center for Big Data & Cloud Computing, He has extended his expertise to explore the cosmos. Through the lens of machine learning, he has uncovered new frontiers in our understanding of the universe, from the meticulous analysis of astronomical data to his contributions to the advancement of space science research.
Talk Title: Machine Learning in Astronomy
Ms. Hira Fatima works as research associate at the Computational Astrophysics Research Lab, NCBC, NED-UET, as research associate. She has served Institute of Space Science and technology, university of Karachi in different capacities for several years. Her research revolves around open and globular star clusters, Milky Way structure and evolution, and Hubble tension.
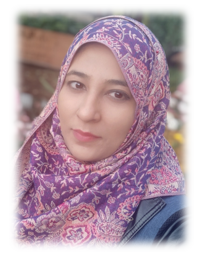
Ms. Hira holds the role of National Astronomy Education Coordinator (NAEC) for Pakistan at the IAU Office of Astronomy Education. Additionally, she serves as the National Coordinator for Pakistan at Astronomers Without Borders. She is approved teacher and exam supervisor for the International Astronomy and Astrophysics Competition. She is also LCO Global Sky Partner 2023.
Talk Title: Starlight and Algorithms: Machine Learning for Open Star Cluster Investigations
Abstract: Delve into the transformative realm of open star cluster research as we explore the revolutionary impact of machine learning. This talk focuses on the paradigm shift in discovering new clusters and precisely determining the membership of known open star clusters using advanced machine learning techniques. Uncover the profound astrophysical and cosmological implications as we harness the power of artificial intelligence to unlock the secrets of open star clusters. Join us on a journey at the intersection of technology and astronomy, reshaping our understanding of the universe.
ORGANIZING COMMITEE
NED University of Engineering and Technology-National Center in Big Data and Cloud Computing, Karachi, Pakistan
Prof. Dr. Saad Ahmed Qazi, Prof. Dr. M. Ali Ismail, and Ms. Hira Fatima
Department of Astronomy, Tsinghua University, China
Prof. Dr. Dandan Xu, Dr. Hongming Tang, Ms. Jiani Chu, Ms. Leyao Wei, Mr. Zechang Sun, Mr. Ce Sui
Radio Galaxy Zoo: EMU Citizen Science Collaboration
Dr. Eleni Vardoulaki, Dr. Hongming Tang
WORKSHOP TENTATIVE PROGRAM
Time Zone: Pakistan Standard Time (PKT) UTC + 05